Learning to Manipulate Anywhere
A Visual Genralizable Framework For Visual Reinforcement Learning
Abstract. Can we endow visuomotor robots with generalization capabilities to operate in diverse open-world scenarios? In this paper, we propose Maniwhere, a generalizable framework tailored for visual reinforcement learning, enabling the trained robot policies to generalize across a combination of multiple visual disturbance types. Specifically, we introduce a multi-view representation learning approach fused with Spatial Transformer Network (STN) module to capture shared semantic information and correspondences among different viewpoints. In addition, we employ a curriculum-based randomization and augmentation approach to stabilize the RL training process and strengthen the visual generalization ability. To exhibit the effectiveness of Maniwhere, we meticulously design 8 tasks encompassing articulate objects, bi-manual, and dexterous hand manipulation tasks, demonstrating Maniwhere's strong visual generalization and sim2real transfer abilities across 3 hardware platforms. Our experiments show that Maniwhere significantly outperforms existing state-of-the-art methods.
Method
We employs a multi-view representation objective to capture implicitly shared semantic information and correspondences across different viewpoints. In addition, we fuse the STN module within the visual encoder to further enhance the robot's robustness to view changes. Subsequently, to achieve sim2real transfer, we utilize a curriculum-based domain randomization approach to stabilize RL training and prevent divergence. The resulting trained policy can be transferred to real-world environments in a zero-shot manner.
Task
To conduct the evaluation, we develop 3 types of robotic arms and 2 types of robotic hands to design a total of 8 diverse tasks.
Tasks in Simulation
Real-world Setup
Our real-world experiments encompass 3 types of robotic arms, 2 dexterous hands, and various tasks including articulated objects and bi-manual manipulation.
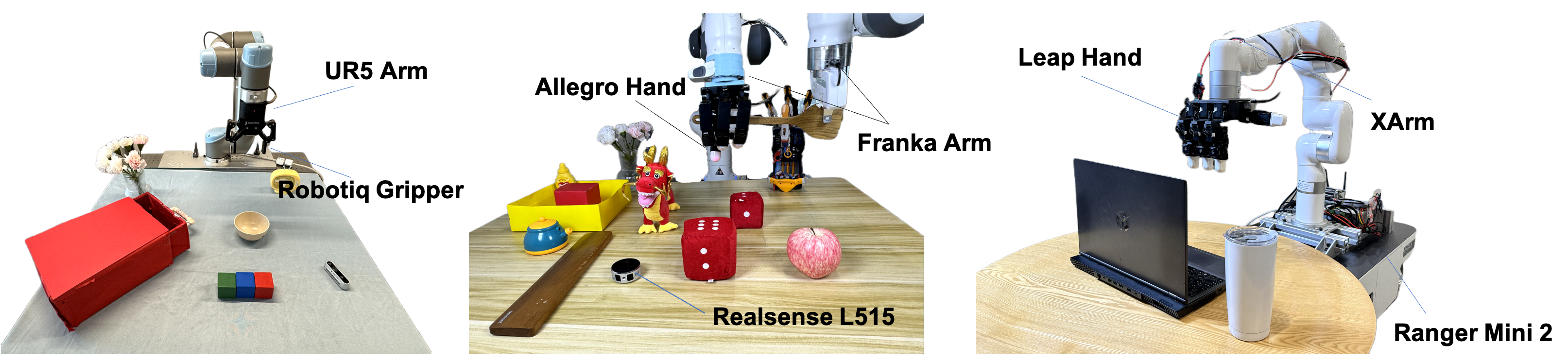
In the following tasks, we directly transfer the simulation trained polices into the real-world in a zero-shot manner. The following videos are recorded from the same view as the policy input images!!
Task 1: Close-Laptop
Task 2: PickPlace Dex
Task 3: Handover
Task 4: PickPlace
Task 5: OpenDrawer
Position Perturbation
Maniwhere can automatically track the position of both pick and place objects rather than acquiring a script policy.
Dynamic Camera Views
ManiWhere is also capable of performing well under dynamically changing viewpoints.
Instance Generalization
Thanks to the general grasping capabilities of the dexterous hand, we find that Maniwhere is not limited to a single object when executing the lifting behaviours and can generalize across different instances with various shapes and sizes.
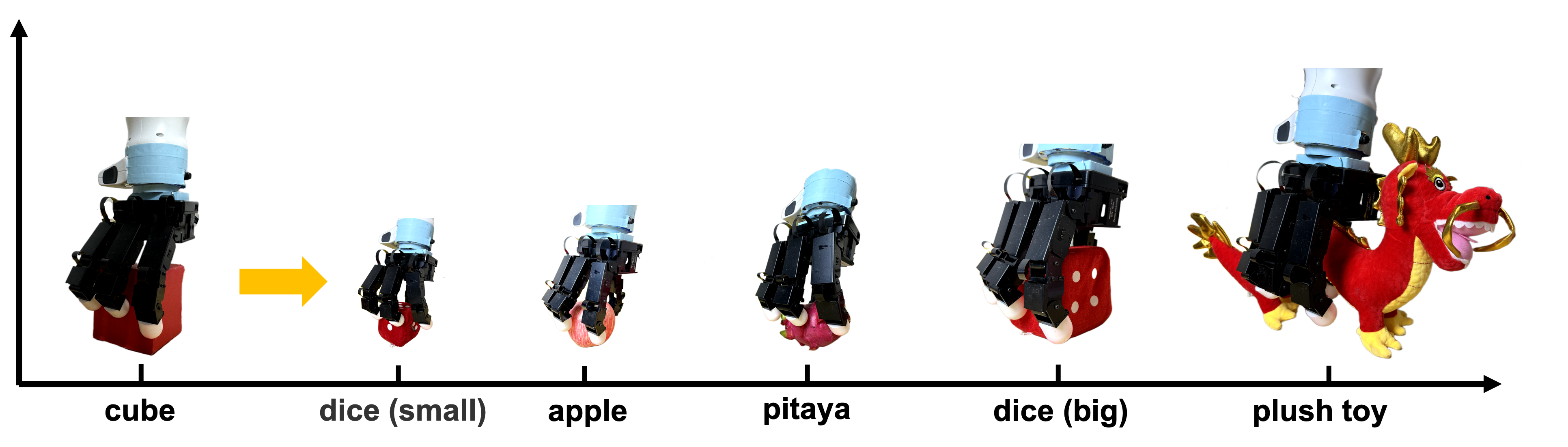
Failure Case
This webpage template is borrowed from DP3.